Dating
You, Girl!
Whether you’re on the hunt for the perfect girl or just want to strengthen your existing relationship, there are a few things to keep in mind. Get help at Dating You, Girl.
What Is
Dating You, Girl
A healthy sexual relationship involves consent, safety, satisfaction, communication and respect. It’s important to remember that everyone is different, and what may feel good for one person may be problematic or damaging to another.
It’s also normal to say no to certain sexual practices if you are uncomfortable with them, as this is your right. But, it’s also not okay to use violence to coerce someone into engaging in a particular sexual practice.
Sexual intimacy is a key component of many relationships and can be a great way to connect with someone. It can include cuddling, oral and manual stimulation and sharing sexual fantasies.
Private HD Live Cams
If you are looking for a great private hd live cams experience, there are many sites to choose from. These sites offer a premium cam model experience with a wide variety of babes who are ready to satisfy your fantasies…
Indian Adult Chat
Indian adult chat is the place where you can meet girls and guys of all ages and interests. You can talk to them in one-on-one or group chats without having to pay. The best part is that you can find…
Sex Webcam History
Sex webcam history is a history of the internet as it has been used to connect people who want to watch sexually explicit acts performed by models. It also serves as a window into a world where economic desperation. Sometimes…
FEATURED STORY
Blind Dating Girls
If a girl feels comfortable around you, it means that she likes you and wants to spend time with you. She may even be flirting with you.
One way to make her feel comfortable around you is to communicate with her in an open and honest manner. When you share stories about your past, she will be able to see you as a trustworthy person who can give her insight into your life.
Another way to build her comfort level is to reveal yourself slowly and gradually. By sharing your personal stories, she will start to feel emotionally attached to you.
Featured Posts
Articles others are reading.
Private HD Live Cams
If you are looking for a great private hd live cams experience, there are many sites to choose from. These sites offer a premium cam model experience with a wide variety of babes who are ready to satisfy your fantasies in HD. There…
How to Meet Girls Online
The Internet is a great place to meet girls online from all around the world. It offers singles of all ages a chance to meet their dream partner in the most efficient way. It is a perfect solution for people who are tired…
Sex Webcam History
Sex webcam history is a history of the internet as it has been used to connect people who want to watch sexually explicit acts performed by models. It also serves as a window into a world where economic desperation. Sometimes it drives some…
Communicating With Her
Communication is a crucial part of any relationship. However, sometimes it can be difficult to convey your needs and desires in a way that your partner understands. This is especially true when it comes to sexual matters.
There are a few key components that go into effective communication, including listening and eye contact. Additionally, it’s important to remember that your girl is a human being with her own unique thoughts, feelings and needs.
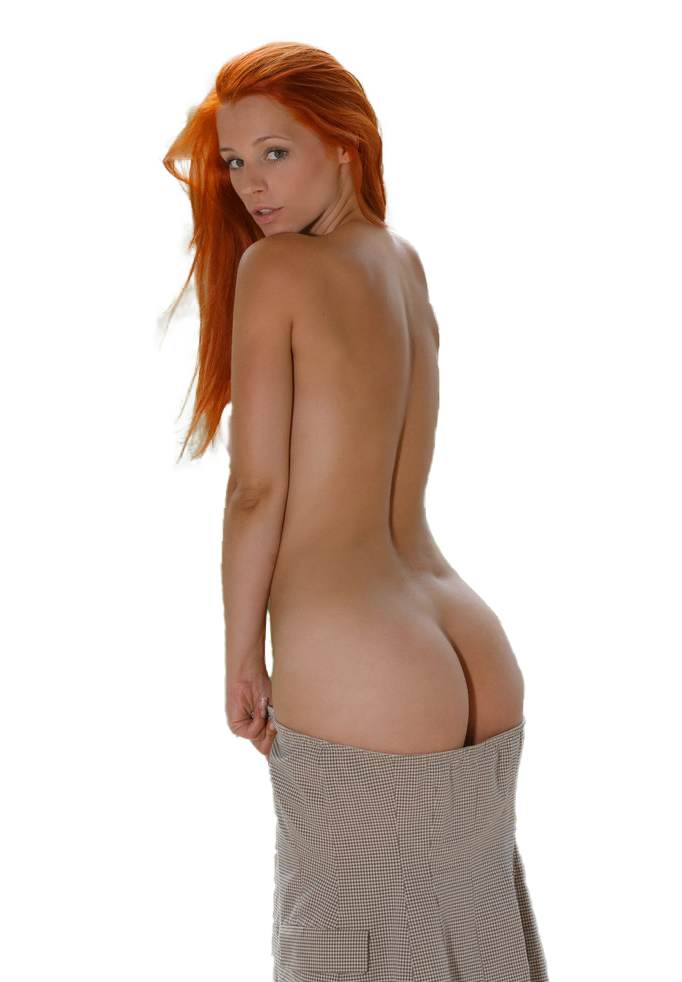